AI-Materials

AI for Modeling of Sustainable Materials
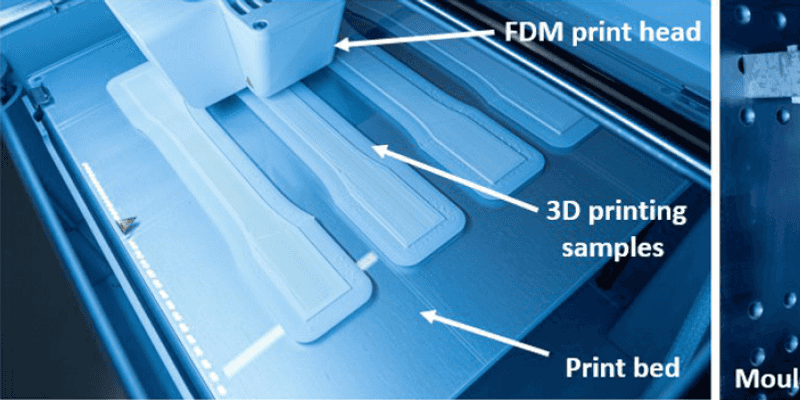
Summary
Modeling of Sustainable Materials. Our research has focused on two central areas: the first one has studied the use of numerical modelling for inference and prediction of the mechanical properties of materials, with special attention to polymers and biopolymers. The second one has been exploring the use of data for improving the design and durability of concrete, our results indicate that the use of machine learning and numerical methods present an advantage when designing new materials with desired properties.
People
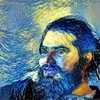
Leonardo – Head of A²I Lab
I am a Principal Lecture in Technology & Head of A²ILab. I have a very broad range of research interests comprehending, but not limited to, areas such as autonomous intelligent machines, mining of big datasets, clustering of time series, causality, quantum machine learning, extreme learning machines, creative machines, philosophy of artificial intelligence, computer vision, deep reinforcement learning among many others.
See profile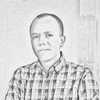
Woubishet – Postdoctoral Researcher
Developing AI models that predict carbonation depth, chloride profile, chloride diffusivity, and hygrothermal performance in concrete structures as well as physical and mechanical properties of stabilized soils.
See profileRecent Posts
Publications 2021
The year 2021 was a great year for the A²ILab. We have published a total of 9 papers, including 2 journal papers, 6 conference papers and…
Read more about Publications 2021Publications
Multitarget regression models for predicting compressive strength and chloride resistance of concrete. Journal of Building Engineering, 72, 106523.. Available at: https://doi.org/10.1016/j.jobe.2023.106523
Characterising the notch root radii and analyses of stress concentration factors near the dominant valleys of rough surface profiles. Rakenteiden mekaniikka, 56(2), 51-61.. Available at: https://doi.org/10.23998/rm.124815
On the Short-Term Creep and Recovery Behaviors of Injection Molded and Additive-Manufactured Tough Polylactic Acid Polymer. Journal of Materials Engineering and Performance, 1-19.. Available at: https://doi.org/10.1007/s11665-023-08278-6
A machine learning method for predicting the chloride migration coefficient of concrete. Construction and Building Materials, 348, 128566.. Available at: https://doi.org/10.1016/j.conbuildmat.2022.128566
Prediction of chloride resistance level of concrete using machine learning for durability and service life assessment of building structures. Available at: https://doi.org/10.1016/j.jobe.2022.105146